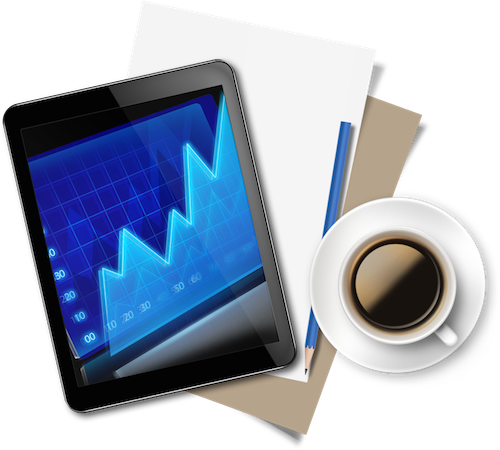
The appropriate time to think about data analysis in not after you’ve obtained a heap of numbers, but before you even begin to collect it. Defining the problem, determining the research design, designing the data-collection tool and deciding the research sample must precede effective data analysis. Proactive thought about the data interpretation sought must be put in before preparing the data-collection tool to ensure that minimum questions yield the maximum result.
Understanding the nature of data is crucial in determining which methods and statistics would be meaningful for your data. Here are the main types:
- Nominal: Represents categories that are mutually exclusive e.g. coding gender where 1 represents male and 2 represents female.
- Ordinal: Represents categories that are rank ordered e.g. school grades where A is exceptional, B is average and C is poor. Differences between categories are not preserved when ordinally scaled.
- Interval: Represents numbers used to rank items such that numerically equal distances on the scale represent equal distances in the property being measured, where the location of the zero point is arbitrary e.g. measures of temperature with the Fahrenheit or Celsius scales.
- Ratio: Represents numbers used to rank items such that numerically equal distances on the scale represent equal distances in the property being measured, where the location of the zero point is fixed.
Before we dive into data
analysis techniques, below is a refresher of some common market research terms.
Already comfortable with this jargon, hop-skip-jump to the next section.
- Population: The total number of measurements or outcomes that conform to some designated specifications relevant to the study e.g. total students at a university.
- Sample: A representative subset of the population used to infer something about the larger group e.g. 50 randomly selected students at the university. The more the variability within a population, the larger the sample size required for more precise characteristic estimation. Sampling designs may be based on nonprobability samples (convenience, judgment, quota) or probability samples (simple random, stratified, cluster).
- Parameter: Characteristic of a population e.g. population mean.
- Statistic: Characteristic of a sample, which may help predict a population parameter e.g. sample mean may predict population mean.
- Outliers: An observation that is so abnormally different in magnitude from other values in the data that the analyst decides to treat it as a special case and may exclude it from analyses e.g. 1 observation with annual household income greater than $900,000, while the remaining 100 data points range between $50,000 to $100,000. An outlier is not an error.
- Hypothesis test: The test to predict a population parameter by rejecting or accepting statistical hypotheses. May be one-tailed or two-tailed depending on the region of rejection. The test aims to verify assumptions while avoiding Type I (alpha) and Type II (beta) errors.
- Independent variables: The predictor variables that are controlled by the researcher to predict the dependent variable e.g. drug dose and time of drug administration.
- Dependent variable: The variable being predicted, which is affected by the independent variables e.g. impact of drug on illness.
- Control: The group within an experiment that does not receive treatment by the researcher and is used as a benchmark against which test subjects are compared e.g. those who did not receive the drug dose.
- Null hypothesis: The hypothesis that is presumed to be true for purposes of statistical testing against an alternate hypothesis. The null hypothesis is rejected based on statistical evidence from the hypothesis test. Usually, the null refers to no treatment effect or no difference between groups e.g. the drug has no impact on treatment of illness. Denoted by H0 it will typically include an equal sign.
- Alternate hypothesis: This hypothesis reflects that there will be an observed effect of the treatment in the hypothesis test. If the null hypothesis is rejected, then we accept the alternate hypothesis. Denoted by Ha or H1 it will typically have an inequality.
Whether it’s just a
handful of numbers or a spreadsheet with countless rows and columns, there’s a
lot you can do to turn data into useful information. Here’s an overview of some
of the most prevalent data analysis methods:
BASIC ANALYSES
- Mean: The average of the data set. It is calculated as the sum of all values divided by the total number of values e.g. average age of a group.
- Median: The value that falls in the middle of the data set when all values are ordered in ascending magnitude. It is equidistant from the smallest and largest values in the set e.g. the fifth value in a set of nine numbers.
- Mode: The value that is repeated most often in a data set. One set may have more than one mode if the frequency of repetition is the same e.g. in the set 8, 9, 9, 11, 11, 14 the modes are 9 and 11.
- Range: The difference between the largest and smallest values in a data set e.g. for a list of random numbers from 10 to 50, the range is 40. The data set should be sorted in magnitude to ensure the largest and smallest values are considered, and not the first and last values.
- Variance: The average of the squared differences from the mean. To calculate, first compute the mean, then subtract the mean from each data value and square the result, and finally work out the average of the squared differences.
- Standard deviation: Measures the amount of variation or dispersion from the average. To calculate, compute the square root of the variance. Represented by the Greek letter sigma.
ADVANCED ANALYSES
- Chi-square test: When understanding differences between groups, chi-square test helps assess the goodness of fit between observed frequencies and those expected theoretically. Typically used when the predictor and outcome are both categorical variables e.g. gender and color preference in apparel.
- t-test: Looks at the difference between two groups on a particular variable of interest, where the independent variables have only two groups. Typically used when the predictor variable is categorical and the outcome is continuous e.g. gender and time spent shopping online.
- ANOVA: Tests the significance of group differences between two or more groups, where the independent variables may be two or more categories. However, the ANOVA (analysis of variance) test only determines whether there is a difference, but does not identify which is different e.g. difference in GMAT scores for different income brackets.
- Correlation: Used to determine the relationship or association between two variables, without distinguishing between independent and dependent variables e.g. price of mobile application and number of downloads.
- Multiple regression: Used to identify the best set of predictor variables among several independent variables and their impact on the one dependent variable e.g. price, quantity and advertising on sales volume. A regression model could also help predict responses to potential changes e.g. impact of price increase by 5%.
- Conjoint analysis: Used to determine the relative preference of different features that make up a product or service e.g. considerations when buying a computer – brand, processor, memory, screen size and price. This helps to understand the tradeoffs made when evaluating several attributes together and possibly identifying opportunities for feature enhancement, modification or withdrawal.
You never know, maybe the reasons for brand switching, customer dissatisfaction, employee turnover and low productivity are sitting in a data set somewhere on your computer. Start the quest to unravel these messages hidden in those numbers.
Subscribe to:
Post Comments (Atom)
Search
Popular Posts
-
Over the past few years, India has seen a sudden mushrooming of startups across various industries, specifically in the technology sector...
-
Facebook has finally allowed us to express in more ways, much beyond the ‘thumbs up’ like button. In September, Facebook CEO M...
-
Searching (often paraphrased as Googling) is an extremely important action on the World Wide Web, growing in double figures each year ...
-
This specially consolidated post of simply explained terminology puts the ultimate digital marketing glossary at your fingertips. Wo...
0 comments:
Post a Comment